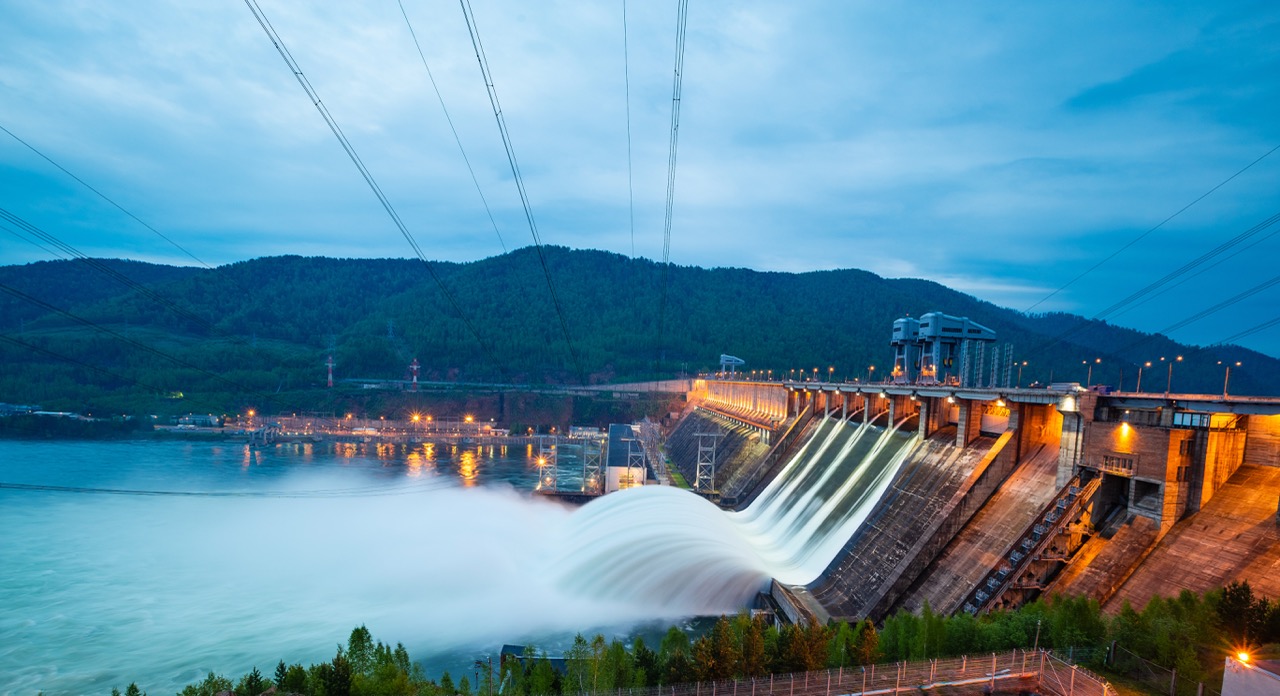
February 12, 2024
February 12, 2024
Better Data Practices in S2S Forecasting: An Industry Perspective
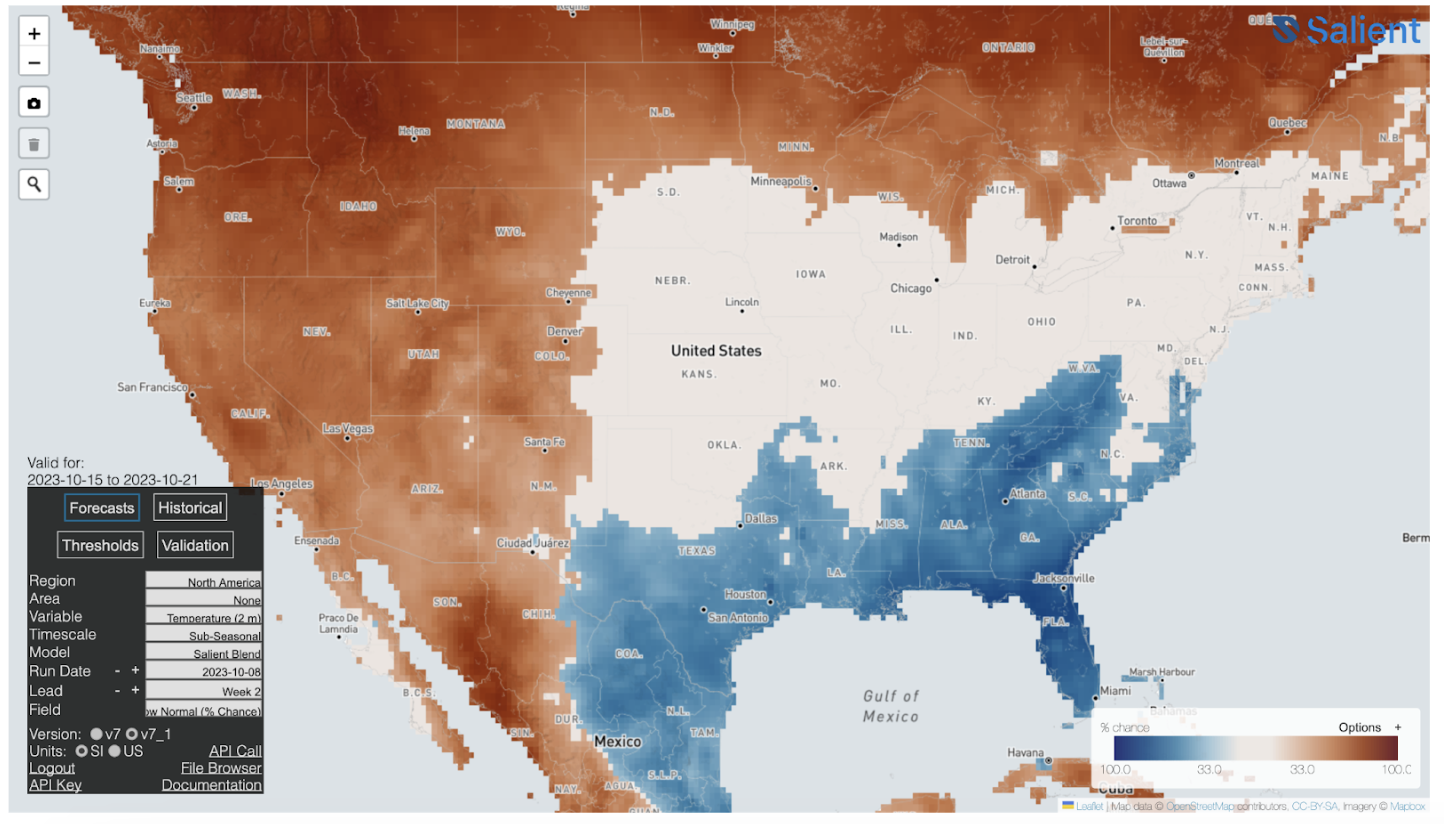
In the evolving field of seasonal-to-sub-seasonal (S2S) forecasting, the Predictability, Predictions, and Applications Interface (PPAI) Panel Webinar Series, hosted by the United States Climate Variability and Predictability Program (US CLIVAR), recently invited Brian Zimmerman, a Climate Scientist at Salient Predictions, to provide insight into how a non-academic entity in the S2S space approaches the problem of S2S forecasting. Zimmerman has an academic background in engineering and climate science but has been working for private industry in the S2S field for the past seven years.
The Challenge of S2S Forecasting
In his presentation, Zimmerman noted the intrinsic challenges of S2S, such as forecast skill and decision-making implementation. Yet interest in S2S forecasting, which represents a decision-critical timeline in large industries like energy, insurance, and agriculture, continues to grow. In recent years, Machine Learning (ML) and Artificial Intelligence (AI) have been employed more frequently as tools to meet these forecasting challenges and create industry solutions. Zimmerman shared how Salient uses AI/ML to enhance S2S forecasts and remain ahead of the curve despite the complexity of data-driven models.
Best Practices: Takeaways
1. Cross-Validation & Scoring
According to Zimmerman, rigorous cross-validation is essential. Salient exemplifies these robust data practices in forecasting and strictly adheres to avoiding data leakage (a common pitfall in ML). The inherent uncertainty involved in forecasting on the S2S timescale necessitates a probabilistic forecast; thus, Salient’s models operate in quantile space, focusing on reliability and proper scoring metrics. Additionally, the validation system within the Salient “stack” allows the team to evaluate skill scores for every metric and model baseline, which allows comparison of each model against each other on all metrics. As a result, the “blending” layer in the Salient stack provides the information it needs to intelligently combine forecasts from different models.
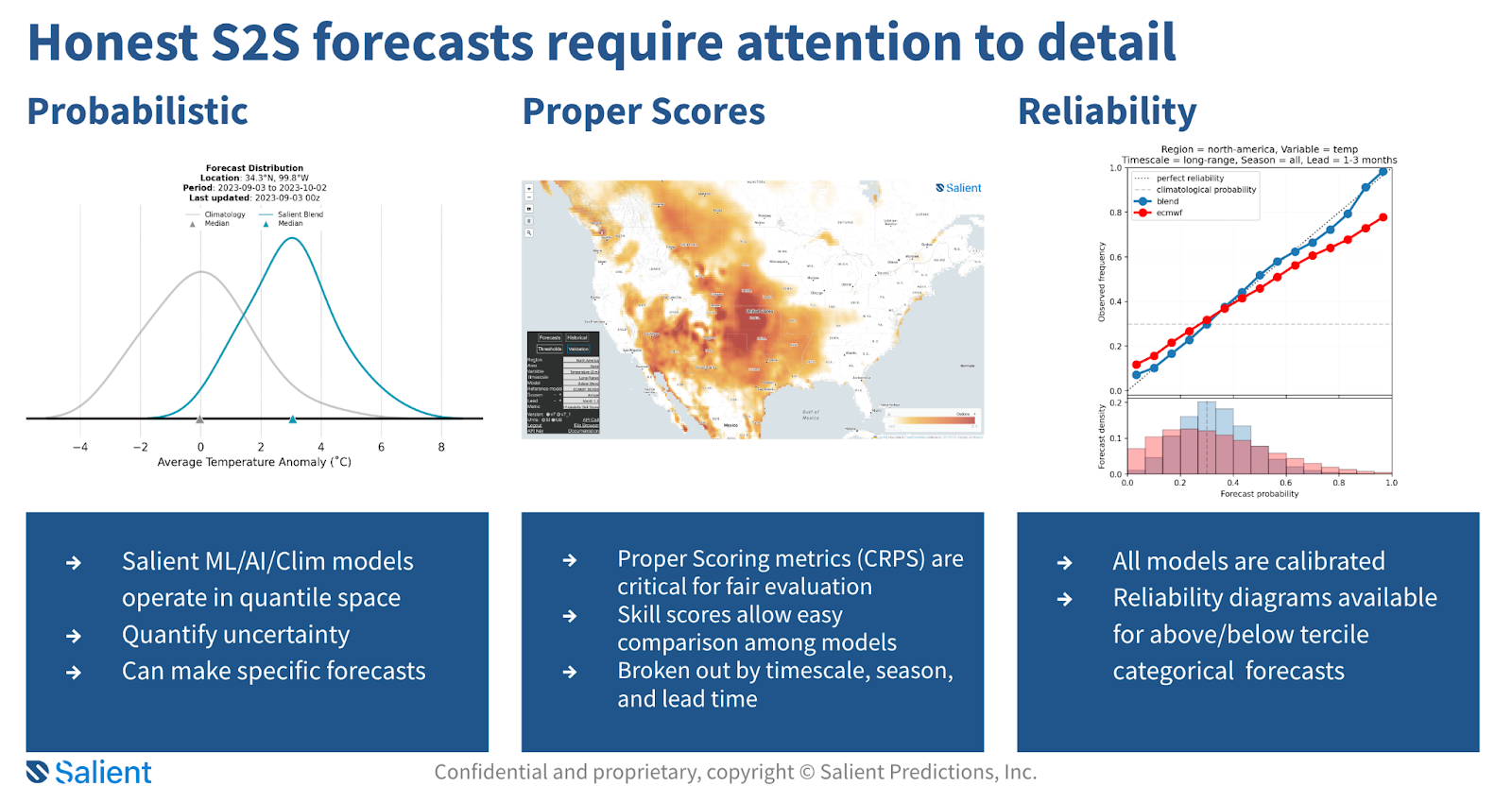
2. Modular Stacking
According to Zimmerman, incisive attention to detail is required to sustain an honest S2S forecast that is reliable and skillful. The team at Salient has thrown all we have into the “Salient Blend” forecast, which comprises a modular stack with various in-house ML models and publicly available dynamical models like ECMWF and GEFS at the bottom layer, calibration models in the middle, and blending models on top. The team’s dedication to continuous refinement of each modular component creates a reliable data-driven forecast, which allows Salient to capture nuanced insights that traditional models might miss.
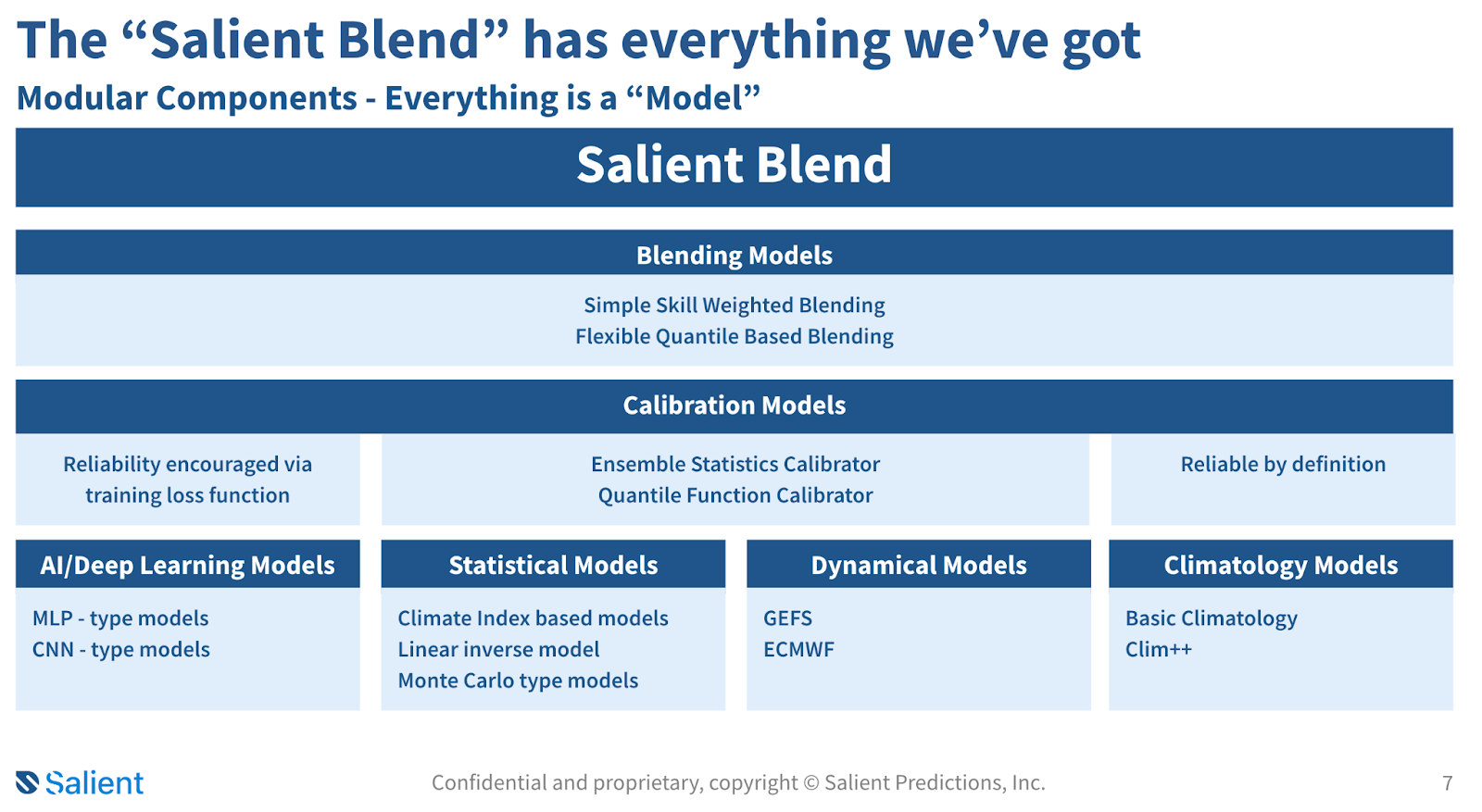
3. Customer-Centric Solutions and a Culture of Discourse
A commitment to both customer-centric and data-driven solutions enables rapid, results-based experimentation. Understanding customers' needs is paramount at Salient, according to Zimmerman, who emphasized the importance of Salient's tailored risk API, which translates forecasts into insights for decision-makers. Salient works hand-in-hand with customers to help them understand the analytics generated by its API and the coinciding value and impact of the forecasts. Iteration designed to meet customer needs means that Salient models are constantly evolving and improving.
Success in S2S forecasting is also dependent on collaboration among a diverse team, from engineers and PhD climate scientists, to machine learning experts and customer success managers. A range of expertise within the Salient team and a culture of discourse keeps the bar high for forecasting best practices. As a result, Salient continues to lead the pursuit of top-tier S2S forecasting solutions.
February 12, 2024
February 12, 2024
Better Data Practices in S2S Forecasting: An Industry Perspective
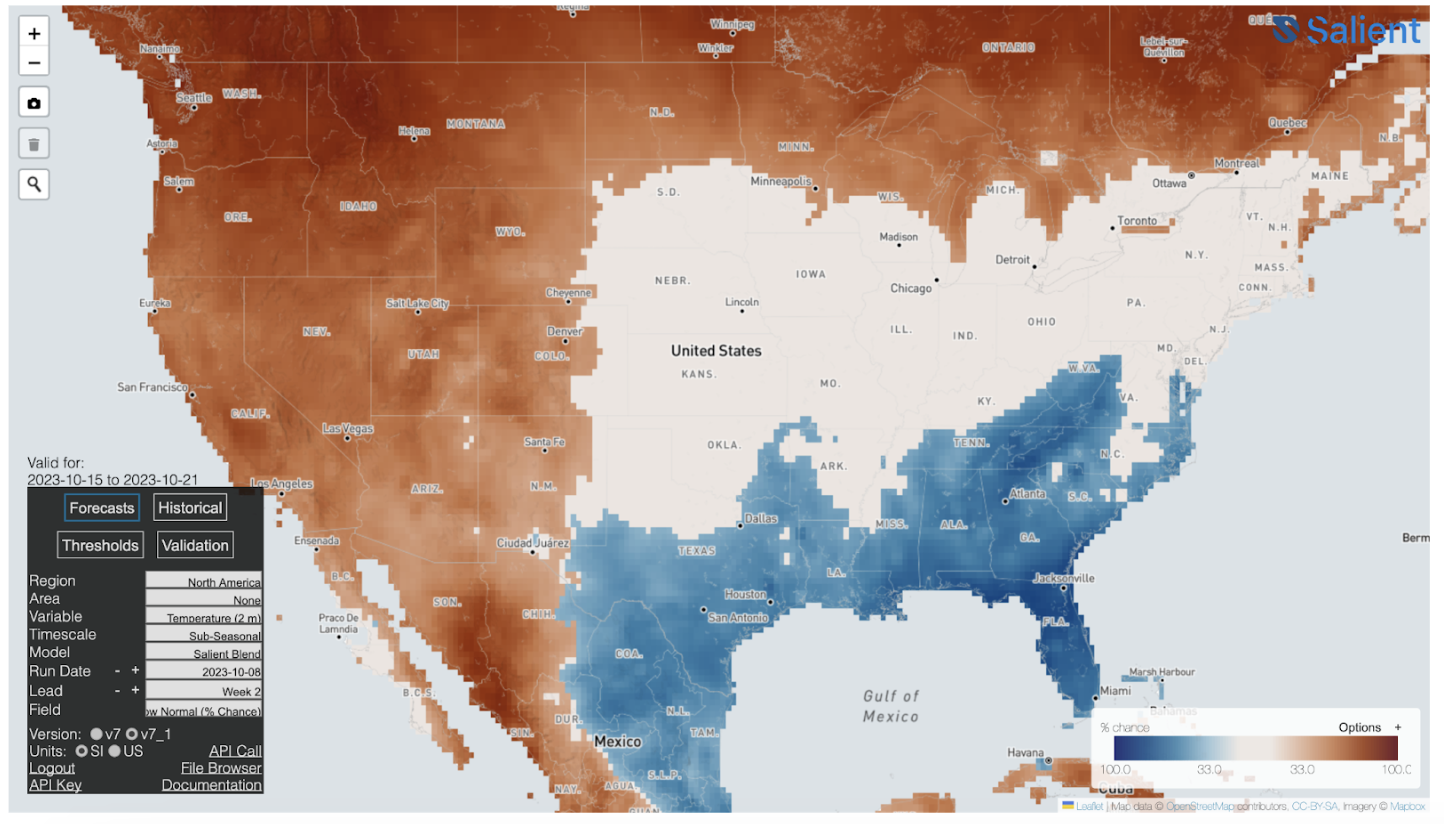
In the evolving field of seasonal-to-sub-seasonal (S2S) forecasting, the Predictability, Predictions, and Applications Interface (PPAI) Panel Webinar Series, hosted by the United States Climate Variability and Predictability Program (US CLIVAR), recently invited Brian Zimmerman, a Climate Scientist at Salient Predictions, to provide insight into how a non-academic entity in the S2S space approaches the problem of S2S forecasting. Zimmerman has an academic background in engineering and climate science but has been working for private industry in the S2S field for the past seven years.
The Challenge of S2S Forecasting
In his presentation, Zimmerman noted the intrinsic challenges of S2S, such as forecast skill and decision-making implementation. Yet interest in S2S forecasting, which represents a decision-critical timeline in large industries like energy, insurance, and agriculture, continues to grow. In recent years, Machine Learning (ML) and Artificial Intelligence (AI) have been employed more frequently as tools to meet these forecasting challenges and create industry solutions. Zimmerman shared how Salient uses AI/ML to enhance S2S forecasts and remain ahead of the curve despite the complexity of data-driven models.
Best Practices: Takeaways
1. Cross-Validation & Scoring
According to Zimmerman, rigorous cross-validation is essential. Salient exemplifies these robust data practices in forecasting and strictly adheres to avoiding data leakage (a common pitfall in ML). The inherent uncertainty involved in forecasting on the S2S timescale necessitates a probabilistic forecast; thus, Salient’s models operate in quantile space, focusing on reliability and proper scoring metrics. Additionally, the validation system within the Salient “stack” allows the team to evaluate skill scores for every metric and model baseline, which allows comparison of each model against each other on all metrics. As a result, the “blending” layer in the Salient stack provides the information it needs to intelligently combine forecasts from different models.
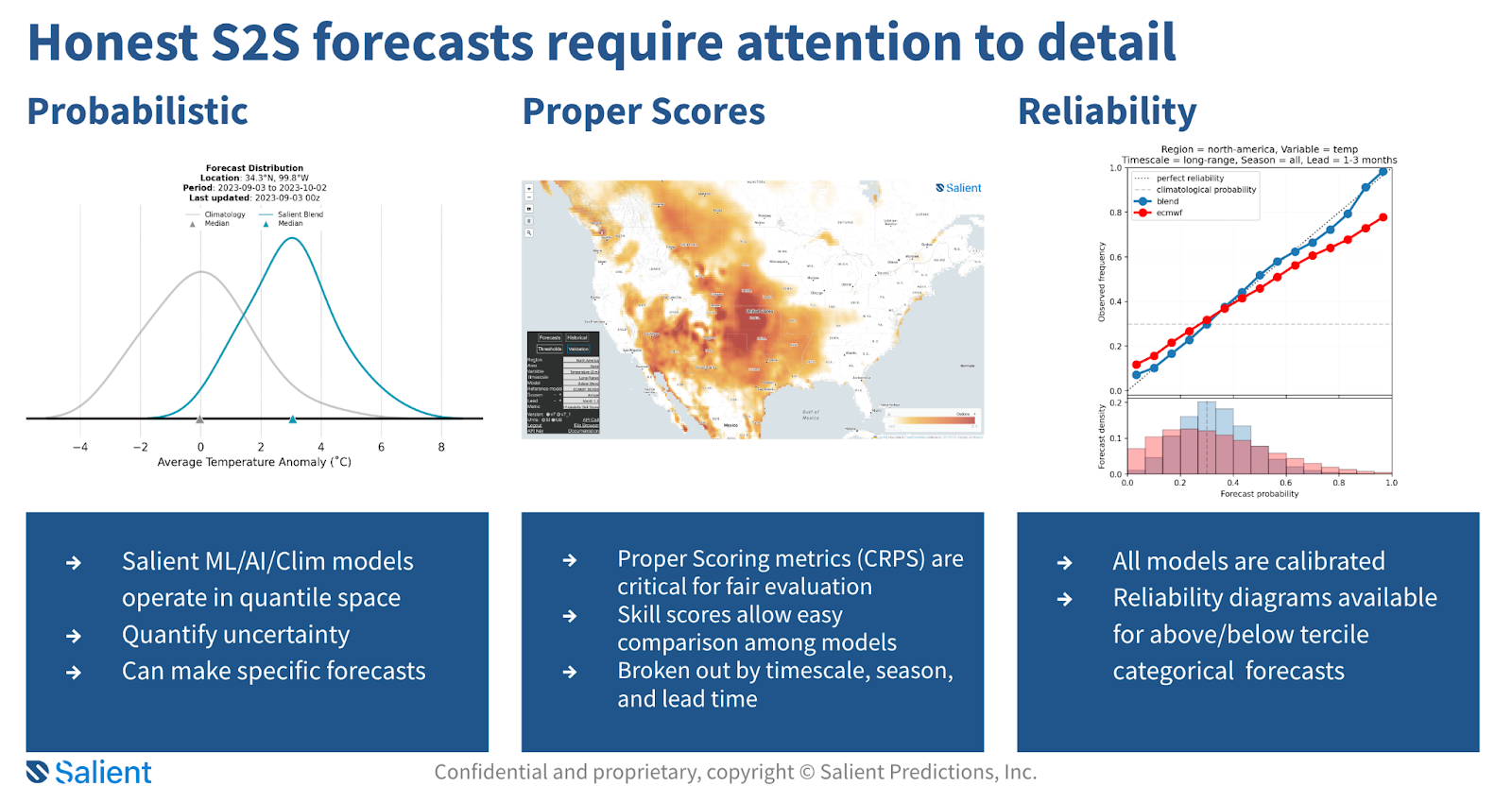
2. Modular Stacking
According to Zimmerman, incisive attention to detail is required to sustain an honest S2S forecast that is reliable and skillful. The team at Salient has thrown all we have into the “Salient Blend” forecast, which comprises a modular stack with various in-house ML models and publicly available dynamical models like ECMWF and GEFS at the bottom layer, calibration models in the middle, and blending models on top. The team’s dedication to continuous refinement of each modular component creates a reliable data-driven forecast, which allows Salient to capture nuanced insights that traditional models might miss.
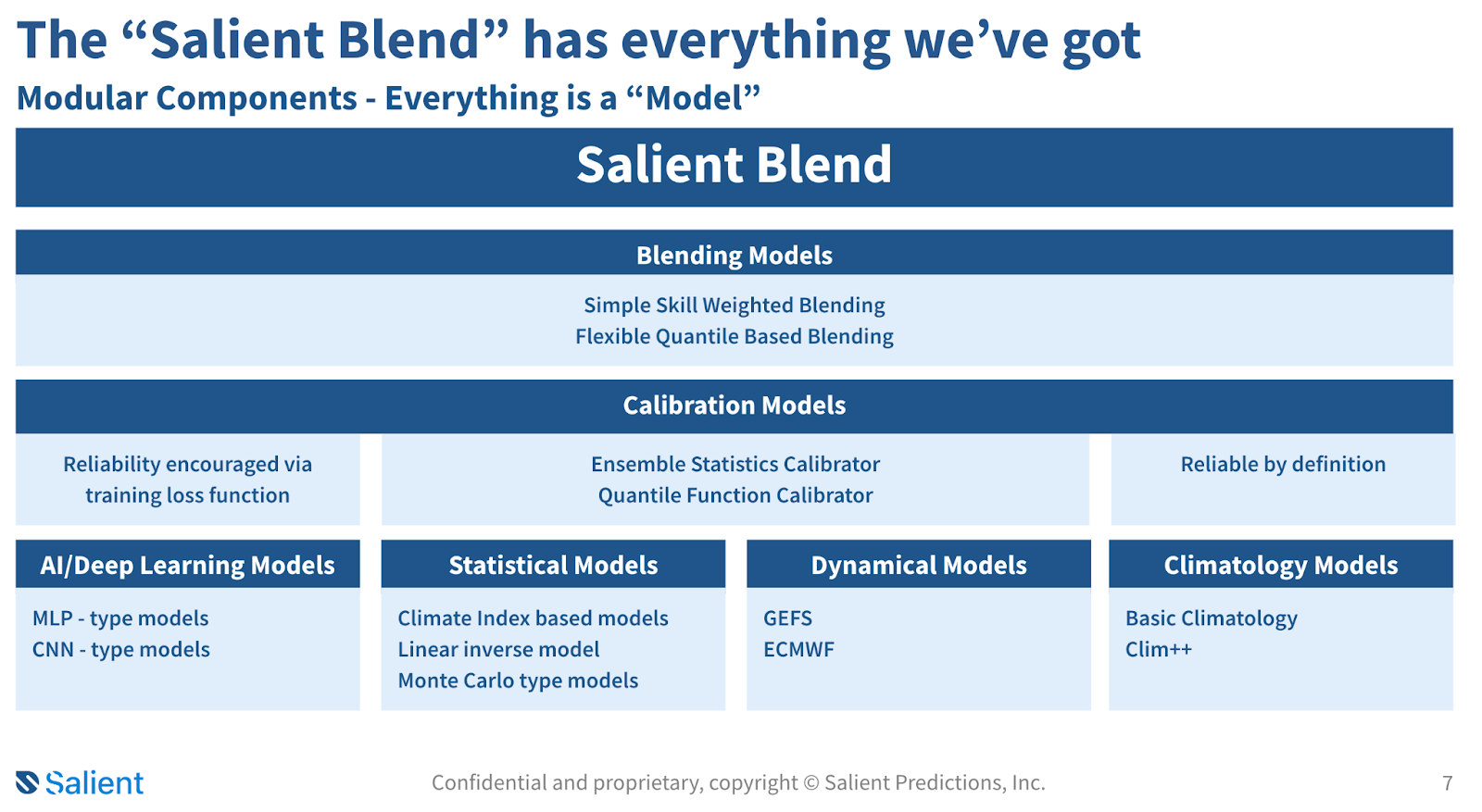
3. Customer-Centric Solutions and a Culture of Discourse
A commitment to both customer-centric and data-driven solutions enables rapid, results-based experimentation. Understanding customers' needs is paramount at Salient, according to Zimmerman, who emphasized the importance of Salient's tailored risk API, which translates forecasts into insights for decision-makers. Salient works hand-in-hand with customers to help them understand the analytics generated by its API and the coinciding value and impact of the forecasts. Iteration designed to meet customer needs means that Salient models are constantly evolving and improving.
Success in S2S forecasting is also dependent on collaboration among a diverse team, from engineers and PhD climate scientists, to machine learning experts and customer success managers. A range of expertise within the Salient team and a culture of discourse keeps the bar high for forecasting best practices. As a result, Salient continues to lead the pursuit of top-tier S2S forecasting solutions.
About Salient
Salient combines ocean and land-surface data with machine learning and climate expertise to deliver accurate and reliable subseasonal-to-seasonal weather forecasts and industry insights—two to 52 weeks in advance. Bringing together leading experts in physical oceanography, climatology and the global water cycle, machine learning, and AI, Salient helps enterprise clients improve resiliency, increase preparedness, and make better decisions in the face of a rapidly changing climate. Learn more at www.salientpredictions.com and follow on LinkedIn and X.